AI Innovations in Drug Discovery
Maya Yadlapalli, Grade 10
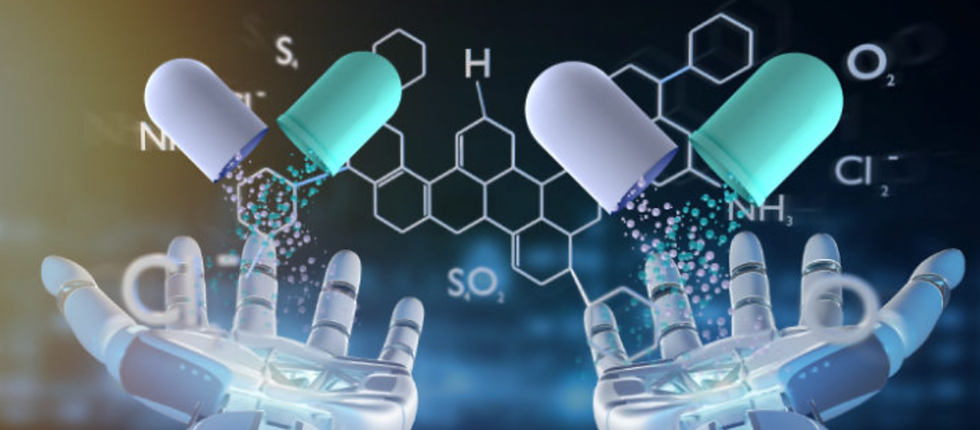
As technology evolves, artificial intelligence (AI) has emerged as a transformative force, influencing nearly every industry. Among its most significant impacts is its role in revolutionizing the medical field, particularly in drug discovery. This technological breakthrough is reshaping traditional approaches, bringing efficiency and precision to a field that has long been plagued by time-intensive and costly processes.
Traditional drug discovery is notoriously slow, often taking 10-15 years to bring a drug to market, with high costs and an equally high failure rate. The challenges stem from identifying drug targets, designing molecules, and ensuring safety and efficacy, all of which require extensive resources. AI is now stepping in to address these bottlenecks, significantly transforming the landscape of drug discovery.
Drug Design and Optimization
AI-driven drug design leverages advanced algorithms like Generative Adversarial Networks (GANs) and reinforcement learning to generate or refine drug molecules. These systems can predict how drugs will interact with their targets, accelerating the development process, reducing costs, and improving precision. By simulating drug interactions computationally, AI reduces the trial-and-error nature of traditional methods, making it faster and more efficient to develop new treatments.
Precision Medicine
AI has also enhanced the field of precision medicine, which tailors treatment plans to individual patients based on their genetic makeup, environment, and lifestyle. This personalized approach improves diagnoses, predicts risks, and optimizes treatment outcomes. For example, cancer immunotherapy and targeted drug therapies, which attack specific cancer cell markers, are real-world applications of precision medicine. These innovations not only advance drug discovery but also improve overall patient care by making treatments more effective and accessible.
Toxicology and Safety Predictions AI is making strides in predicting the toxicity of drug candidates early in the development process, reducing failure rates, and minimizing reliance on animal testing. Models trained on historical toxicity data can flag potential issues before costly clinical trials. One notable example is MIT’s AI model for toxicity prediction, which has demonstrated significant promise in this area, saving time and resources while ensuring safety.
In conclusion, AI has drastically reduced the time, cost, and uncertainty associated with drug discovery. Streamlining processes, minimizing trial-and-error, and personalizing treatments, it is reshaping how we approach medicine. While concerns about AI's misuse remain valid, its potential to improve human life when used responsibly is undeniable. Harnessing AI's power ethically and thoughtfully will continue to drive groundbreaking advancements in healthcare, benefiting patients and practitioners alike.